Could AI Super-Charge your Sustainability Programmes? What are the Pros and Cons?
AI has a Marmite rep. You like it or you don't. It is both the unknown and the fear of the unknown. Where and how can AI be used to deliver sustainability projects?
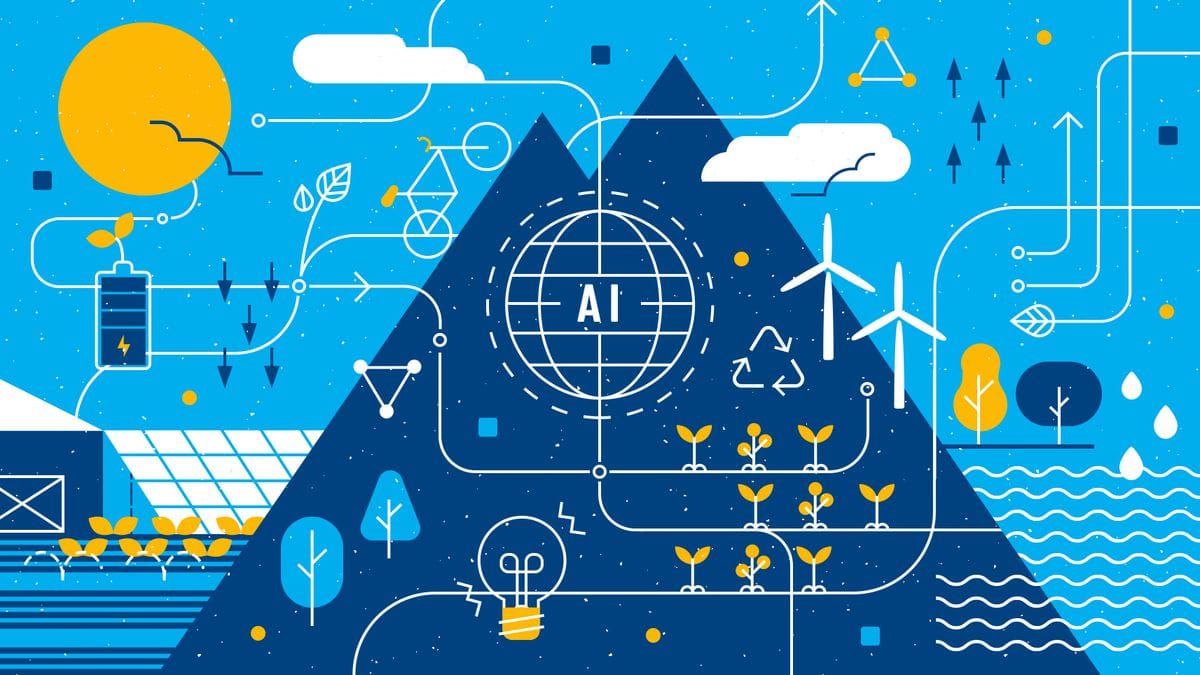
AI has a bad rep. It is both the unknown and the fear of the unknown. Can we harness AI to benefit sustainability drives positively?
Companies adopting net-zero targets are focussing on reducing direct and indirect emissions and many are employing AI to manage and mitigate that environmental impact.
A typical net-zero plan involves measuring and reporting emissions, optimizing operational processes to reduce emissions, and offsetting residual emissions through various initiatives.
AI can play a significant role in enhancing corporate sustainability efforts across various industries. But there are legitimate concerns. While AI offers significant potential in advancing sustainability programs, there are also controversies and concerns associated with its implementation. Some of the key controversies include:
Energy Consumption of AI Systems: Training and running complex AI models can require substantial computational power, leading to high energy consumption. This irony, where AI is used to address sustainability challenges but contributes to increased energy consumption, has sparked debates about the overall environmental impact of AI.
E-Waste and Hardware Production: The rapid evolution of AI technology leads to frequent upgrades and replacements of hardware components. This contributes to electronic waste (e-waste) and raises concerns about the environmental impact of manufacturing, disposing of, and recycling AI-related hardware.
Data Privacy and Security: AI systems often require large datasets for training, and the collection and handling of this data can raise privacy concerns. In sustainability programs, especially those involving consumer behavior analysis or supply chain tracking, ensuring the privacy and security of sensitive information becomes crucial.
Bias and Fairness: AI models can perpetuate and amplify biases present in the training data, leading to unfair and discriminatory outcomes. In the context of sustainability, biased algorithms may disproportionately impact certain communities or contribute to environmental injustice.
Lack of Regulation and Standards: The rapid development of AI technology has outpaced the establishment of comprehensive regulations and standards. This creates challenges in ensuring the ethical and responsible use of AI in sustainability programs.
Dependency on Technology: Relying heavily on AI for sustainability solutions may lead to a dependency on technology, potentially overshadowing other essential aspects of sustainability, such as community engagement, policy changes, and behavioral shifts.
Short-Term vs. Long-Term Trade-offs: Some argue that while AI may offer short-term gains in efficiency and resource optimization, it may inadvertently lead to long-term consequences if not carefully managed. For instance, focusing on immediate cost reductions through AI may overlook broader ecological and societal considerations.
Job Displacement Concerns: The automation capabilities of AI raise concerns about job displacement, particularly in industries where AI is implemented for efficiency gains. This socioeconomic impact could have implications for the communities and individuals affected.
Algorithmic Accountability: As AI systems become more complex and autonomous, there is a growing need for transparency and accountability in their decision-making processes. Understanding how AI systems reach conclusions in sustainability programs is crucial for trust and ethical considerations.
Addressing these controversies requires a comprehensive and multidisciplinary approach involving stakeholders from technology, policy, ethics, and environmental fields. Striking a balance between harnessing the benefits of AI for sustainability and mitigating its potential drawbacks is essential for responsible and effective implementation.
OK, but give me examples where AI helps sustainability programmes
Emissions Monitoring and Reporting: AI can analyze and monitor a company's emissions data, helping identify areas for improvement. This allows businesses to measure, report, and manage their carbon footprint more effectively.
Supply Chain Optimization: AI can be employed to track and analyze the sustainability practices of suppliers. This helps companies make informed decisions about their supply chain, choosing environmentally friendly and socially responsible partners.
Predictive Maintenance: AI can predict equipment failures and schedule maintenance, reducing unscheduled downtime and preventing unnecessary energy consumption. This contributes to more efficient resource use and a lower environmental impact.
Smart Transportation: AI can optimize transportation networks and logistics, minimizing emissions from transportation by finding the most efficient routes and scheduling deliveries strategically.
Energy Efficiency: AI can optimize building energy systems, lighting, HVAC systems, and other equipment to reduce energy consumption. This leads to cost savings and a decrease in carbon emissions.
Renewable Energy Integration: AI can help integrate renewable energy sources, such as wind and solar, into the grid more efficiently. This ensures a higher proportion of clean energy in the overall energy mix.
Carbon Capture and Storage: AI can optimize the design and operation of carbon capture and storage systems, contributing to the reduction of emissions from industrial processes.
Demand Forecasting and Inventory Management: In sectors like retail and manufacturing, AI-driven analytics can predict demand more accurately, leading to optimized inventory management and reduced waste.
Consumer Behavior Analysis: AI can analyze consumer behavior to provide insights into preferences and trends, helping businesses tailor their products and services to be more sustainable and aligned with customer values.
Collaborative Research and Development: AI can facilitate collaborative efforts between companies, research institutions, and government bodies to innovate and develop sustainable technologies and practices.
AI can contribute to achieving corporate sustainability goals, aligning business practices with broader environmental and social objectives. It is not the ‘be all and end all’, and sustainability leaders need to carefully analyse where AI would be useful, and where staff time would be better deployed. By selectively incorporating AI technologies in these ways, companies ‘could’ make their operations more environmentally friendly, economically efficient, and socially responsible. ‘Could’! Choosing the right partner or service provider, as ever, is key.